Documentation
Case for Support
Introduction
This research will fundamentally transform our understanding of daily urban movement patterns through the marriage of ‘big data’ and cutting-edge computer simulation. It will develop new methods to produce data that will help us to address key issues in crime and health.
A big data “revolution” is underway that has the potential to transform our understanding of daily urban dynamics and fundamental approaches to quantitative social science (Savage and Burrows, 2007; Mayer-Schönberger and Cukier, 2013). Vast quantities of new data are being gathered in cities. New services are capturing information about the daily actions of individuals from their use of social media (Stefanidis et al., 2013), public transport systems (Seaborn et al., 2009) and mobile telephones (Ratti et al., 2006), to name a few. Data from these sources, although noisy, messy and biased are unprecedented in their scope, scale and resolution. The research will first develop new geospatial methods that can make sense of these data and derive information about peoples’ daily spatio-temporal behaviour. It then proposes a novel concept: to develop a computer simulation of individual-level, city-wide daily urban movements that will be calibrated dynamically from streams of crowd-sourced data. This combination of big data and simulation will both resolve many of the drawbacks present in individual data streams – in particular the inherent bias and noise – and also create insights into daily urban movements that will be ground breaking in their spatio-temporal resolution and individual detail.
This research is original and important because previous attempts to model detailed urban movements have been hampered by a lack of high-resolution data and by methods that have difficulty in accounting for the complex individual-level interactions that ultimately drive the urban system (Batty, 2007, 2012). Large-volume sources, such as censuses, capture attributes and characteristics of the population, rather than their attitudes and behaviours (Malleson and Birkin, 2012; Birkin et al., 2013). Conversely, detailed surveys that attempt to capture this behavioural information are naturally limited by their size and scope. In contrast, new ‘big’ data streams are voluminous and contain information about a user’s location as well as a textual or multimedia component that often describes their behaviour or actions (Crooks et al., 2013). The new simulation model will make use of these data to create a picture of urban dynamics that will be unparalleled in its spatio-temporal resolution.
A clearer picture of urban dynamics will have the capacity to alter our understanding of key social phenomena that depend on the locations of mobile populations. This research will use the simulation outputs to generate new population at risk estimates – based on peoples’ daily travel behaviour rather than their residential location – and apply these estimates to two empirical areas:
-
Crime – a reappraisal of crime hotspots based on accurate estimates of the potential victims rather than simply the residential population;
-
Health – an analysis of peoples’ exposure to air pollution based on where they actually spend their time, rather than where they live.
Closely aligned to the ESRC strategic priority of influencing behaviour and informing interventions, the research will use the new model of urban dynamics to better understand key social phenomena and explore the ways in which interventions can be developed to inform individual behaviour and ultimately improve societal wellbeing. The project will build on both the PI’s world leading simulation expertise (Malleson et al., 2013) as well as his recent research into big data and social media (Birkin et al., 2011) and then expand into broader areas. International visits and subsequent collaboration will help to construct a world-leading team of researchers positioned around the PI’s research interests. Future Leaders funding will provide the time and resources for him to become a distinguished academic in this important, highly topical field.
Research Questions
This research will employ advanced quantitative methods for the analysis of new sources of data, followed by the application of computational modelling to build a new conceptual picture of urban movement dynamics which will be applied to areas of practical societal relevance. The research questions are:
-
What are the computational methods required to harness, manage and evaluate social ‘big data’ to inform our understanding of popular movement patterns?
- How can computer simulation be used to combine data from diverse sources, reduce biases, and create a new conceptual picture of daily urban dynamics?
-
How should crime-reduction initiatives be updated to reflect a new understanding of crime risk, derived from a clearer picture of the spatio-temporal locations of crime victims?
- What are the true levels of exposure to polluted air, based on individuals’ actual daily behaviour rather than their residential location, and how do these impact national health?
Research Methods and Data
Research of this type poses a number of ethical questions, particularly around the issues of informed consent, privacy, surveillance, anonymity and data security. These are not, by any means, unique to this project – all ‘big data’ and ‘smart cities’ initiatives will make similar considerations. To mitigate the risks this project will take steps to ensure that all individual-level data are adequately anonymised and, as with all research at the University of Leeds, will undergo stringent ethical review. For full details see the Ethical Information section and Data Management Plan. The PI and mentor both have experience in working ethically with these types of data and previous projects have already undergone internal ethical review. The project will also be able to follow best practice guidelines from new big data and smart cities projects that have been recently initiated by the ESRC and the Government.
The project has been divided into three work packages (see Figure 1 for an overview). These focus on analysing ‘big’ social data, producing a simulation model and then generating new population at risk estimates that can be applied to areas of substantive social interest.
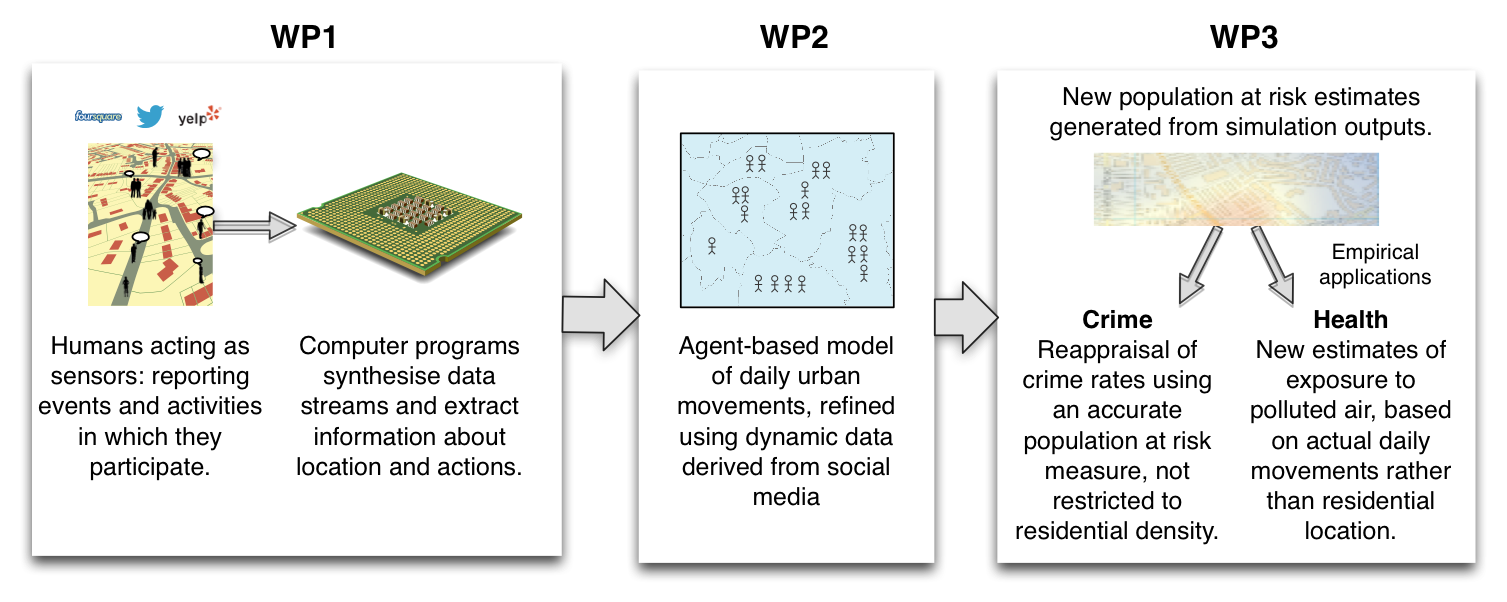
WP1 – Harnessing Big Data and Inferring Behavioural Information
The emergence of social media and other digital services means that people often act as sensors; they report events and activities in real time. This work package will begin by developing software that can synthesise data from these services and deduce popular movements. Initially, Twitter, Yelp, Foursquare and Flickr will be used. Each service provides public access to their data streams through application programming interfaces (APIs). Additional sources that can be used include records of public transport use (e.g. the Oyster card in London), the locations of mobile telephones and other social media services that will emerge over the course of the research. The PI already has experience in collecting these types of data (Birkin et al., 2013). Traditional sources (such as the 2011 UK Census and Understanding Society) can be used to estimate, albeit at a lower resolution, the movement patterns of groups of people who do not use social media. Although many new, ‘big data’ sources will exclude large sections of society, this research aims to limit these biases by combining data from various sources, including aggregate surveys like the Census. Furthermore, the PI is also fostering a working relationship with Telefónica UK (the owners of the O2 mobile phone company) who have provided a letter of support. Through this collaboration the research will have access to a database describing the locations of mobile phone hand sets each time they communicate with the network. Although all data will be anonymised, data security and ethical implications are principal concerns with these sources. The Data Management Plan outlines the data security procedures that have been put in place and ethical implications are addressed comprehensively in the Ethical Information section. This proprietary data will provide the opportunity to engage groups who do not participate in the forms of communication mentioned above, partially resolving one of the biggest project risks.
In addition to reading data streams, the substantive activity in WP1 will be to develop computational methods to extract useful information about individual movements from the data. Preliminary work conducted by the PI (Malleson and Birkin, 2013), has identified regularly visited areas (using Kernel Density Estimation on individual message locations) and used regularly occurring words to identify the function of an area (e.g. home, work, etc.). This work will be extended to identify clusters of activity in time as well as space, using existing cluster hunting routines such as ST-DBSCAN (Birant and Kut, 2007) and SaTScan (Kulldorff, 2001). The application of these algorithms to social media data to estimate behaviour has yet to be attempted.
WP2 – Developing and Optimising A Model of Urban Dynamics
Developing an accurate understanding of urban activity patterns has been hampered by limited high resolution data and by modelling methods that work at an aggregate spatial scale. This work package will centre on the development of an agent-based model (ABM) of individual human activity patterns, refined using the information about daily population movements from WP1. The main advantage with the methodology is that models are able to encapsulates system-wide characteristics by simulating the behaviour of individual ‘agents’ (people in this case) directly, rather than by attempting to derive aggregate equations for system dynamics (Bonabeau, 2002). For this project, an ABM will be developed that replicates the daily behaviour (e.g. working, shopping, leisure activities, etc.) of individuals in a city, effectively creating a virtual representation of the urban system. The PI has had ongoing success applying the technique to the study of social systems (Malleson et al., 2010, 2012, 2013).
To create an initial population of individuals for the agent-based model, microsimulation will be used to disaggregate the 2011 UK Census into distinct individuals and household units. This technique is well established (Harland et al., 2012; Malleson and Birkin, 2012). An important element of the work will be the development of methods to adjust the behaviour of the individual agents to match the that identified from dynamic ‘big’ data streams (i.e. model calibration). This will be accomplished by first identifying clusters of behaviour in the data – such as people from a given area regularly travelling into the city centre for work at a particular time – and using these to adjust the behaviour of the simulated individuals. Existing approaches to dynamic data-driven simulation, such as Kalman filters or Sequential Monte Carlo methods (Xiaolin, 2011) can potentially be adapted for use; they are commonly used in the physical sciences but insufficient dynamic data streams have limited their use in social simulation. Hence their adaptation for an agent-based model to calibrate human behaviour from dynamic social data streams will be an exciting and important development for the field.
WP3 – Applications to Social Phenomena
The final work package will focus on delivering impact through the application of the new model of urban dynamics to two areas of substantial social importance: crime and health. These application areas are substantively different which demonstrates the robustness of the methods across multiple domains. They will allow the PI to build on existing crime analysis expertise (Andresen and Malleson, 2013) and develop new proficiencies that will broaden his skill base and help to build networks with academics in other fields. Accomplishing both applications, on top of data analysis and model building work, will be challenging. Hence the second project (health) will be a small preliminary study that can later be expanded into a full funding proposal (see the Workplan for details).
The first project will focus on the analysis of patterns of crimes against mobile victims (e.g. street crime, robbery, etc.). It is well known that inherent differences in their underlying causal mechanisms mean that the rates of different types of crime require different denominators to measure the population at risk (Boggs, 1965). Most research uses the residential population which is unsuitable for crimes that involve mobile victims such as as assaults (Boivin, 2013), robbery (Zhang et al., 2012) and violent crime (Andresen, 2011). This study will use common spatio-temporal cluster hunting techniques (for examples see Kulldorff, 2001) to identify clusters of crime using local and national crime data. The novel and important aspect of the work will be the use of a population at risk measure, derived from the urban simulation, that is both spatio-temporally accurate and optimised for the type of crime. Although this has been recognised as vital for an accurate understanding of crime rates for some time (Boggs, 1965), crime research has yet to make such a breakthrough.
The second project, an exploratory analysis, will reappraise the means by which air pollution is associated to personal exposure and subsequently to health. Prior research has developed comprehensive spatio-temporal estimates of air quality in the Leeds area (Mitchell, 2005). Crucially, however, exposure to polluted air is estimated using residential locations that are clearly unsuitable for populations whose routine activities regularly draw them away from home (e.g. for work, leisure, etc.). This project will couple air quality predictions to dynamic estimates of individual behaviour to properly measure exposure. It is likely that actual exposure is being significantly under-estimated at present which will have impacts on national/EU air quality legislation and has the potential to fundamentally transform our understanding of the impacts of air pollutio on public health.
Expected Outputs and Impact
To disseminate this research to the international academic community, a methodological paper will be submitted to a leading geography or social science journal (such as The International Journal of Urban and Regional Research) and two empirical papers (originating from the case studies) will be sent to the highest impact, most relevant journals (such as Quantitative Criminology or Atmospheric Environment). As well as presenting at major international conferences, the PI will develop special sessions at two conferences that he is organising: GISRUK in 2015 and the European Colloquium on Theoretical and Quantitative Geography (ECTQG) in 2017. Also, a course will be organised to run as part of the locally hosted ESRC NCRM node to train postgraduates in the advanced quantitative analysis of ‘big’ social data and associated methods for social simulation. As an active member of the Leeds Social Science Institute (an organisation that aims to coordinate the activities of social scientists across the University) steering committee, the PI is in a strong position to disseminate the research to other social scientists in the University and, through his involvement in the Worldwide Universities Network (WUN) and with overseas visits, to an international audience. The research will also generate outputs for non-academic users (produced in collaboration with a user working group) and work with the University press office to elicit the greatest impact from project outputs. To build an online presence, the project will create a website and also an online portal that will provide access to software codes and ongoing results. Funds are sought to appoint a Research Associate whose duties will include building these online applications with which to engage users.
The potential impacts of this project are threefold. Firstly, the project will contribute vital empirical and methodological innovation to the growing body of research around the concept of big data and smart cities. This is an area that has been recognised as highly important by the ESRC – who have recently allocated 64 million in capital funding – and by the Government – who have recently set up the Future Cities Catapult and recognise big data as an area in which Britain could be a “global leader” (Willetts, 2013). This research aims to leverage resources provided by these new investments, potentially as a consumer of the new data. This will help to capitalise on and add value to ongoing ESRC investment. The host institution is actively participating in these funding competitions and the PI has been contributing to Leeds’ bids. Secondly, the project will develop innovative approaches to dynamic model optimisation that will be vital if the field of social simulation is going to reduce the methodological gap with counterparts in the physical sciences that routinely make use of dynamic data streams in models (Collins, 2007). Thirdly, it will offer substantial strategic benefit to policy makers and public/private organisations who require greater knowledge about dynamic urban movement patterns. In particular, the research will allow crime reduction practitioners to re-assess crime hotspots armed with new knowledge about the possible victims and it will impact on clean air legislation once a better understanding of actual exposure has been developed (see the Impact Statement for details).
Institution, Mentor and International Networking
The research will be hosted by the Centre for Spatial Analysis and Policy (CSAP) in the School of Geography, University of Leeds. The School of Geography is in the top 20 QS World Ranking and CSAP is one of the most respected centres in the world for the application of quantitative geographical techniques to the study of social phenomena. The Centre has pioneered new computational modelling and data analysis methods that are directly relevant to the proposed research, including spatial microsimulation, spatial interaction modelling and geographical clustering. The work of CSAP also has a strong empirical focus and links with the private and public sectors (in organisations such as Asda-Walmart, Acxiom, West Yorkshire Police and Leeds City Council) will help to ensure research impact beyond academia.
Professor Birkin, the applicant’s mentor, has an extensive track record in developing quantitative geographical methods that are directly relevant to this research as well as a substantial portfolio of 1M+ research projects (including a current ESRC NCRM node). He has extensive international connections in geocomputation, social simulation and regional science. The PI regularly publishes with Prof. Birkin (Birkin et al., 2011, 2013; Malleson and Birkin, 2012, 2013); demonstrating an alignment of research interests but without a student/supervisor relationship. Importantly, the project will also allow the PI to take advantage of networking opportunities to begin building a research group around his interests. Nationally, the PI will build on existing links with the Centre for Advanced Spatial Analysis (University College London) and other groups conducting ‘big data’ research such as the Open Data Institute (Southampton), LSE Cities (London), the Institute for Future Cities (Glasgow), and others that will emerge over the course of the research. Internationally, the PI will seek to build new links with the Senseable City Laboratory at MIT through a funded research visit (mentored by Carlo Ratti). The Laboratory are world leaders in urban dynamics and the analysis of big data, so such a collaboration will be crucial to the success of the research as well as to the PI’s international networks. A short visit to the Institute for Canadian Urban Research Studies (ICURS) at Simon Fraser University in Canada will support the crime case study. The Institute is directed by Profs. Brantingham who are seminal voices in the field of environmental criminology and are ideally placed to support the theoretical basis of the applied work. In addition, links with Centre the for Social Complexity (George Mason University) will be exploited to build on their relevant work in harvesting and analysing social media data (Crooks et al., 2013). The PI has recently submitted a 290k grant under the Digging Into Data call with Dr Crooks and colleagues.
Programme of Skills Development
Academic Leadership
Even at this early career stage, the PI is building a reputation as a talented researcher. He already has a portfolio of papers published in high impact journals, has managed a successful JISC grant, obtained funding for PhD projects, sits on the Leeds Social Science Institute and Security & Justice management boards, organised local research groups and has chaired/organised sessions at international conferences such as the Association of American Geographers, the Royal Geographical Society Annual Conference and the International Geographical Union. Leading this research project will provide the resources, experiences and time for the PI to build on these accomplishments and become an academic leader.
To this end, future leaders funding will be used to develop academic leadership skills in the following ways. The visits to world-leading institutions will provide the opportunity to begin building a network of researchers around the PI’s research interests which will demand new leadership skills. Building on previous experiences organising sessions, the PI will organise two conferences in Leeds in 2015 and 2017. The management of a larger, longer-term project and with the mentoring of an RA will also help to broaden this experience and will require formal skills development. Formal leadership training will be conducted through training courses run by the Staff and Departmental Development Unit (SDDU) at Leeds. These will include, among others, ‘Introduction to the Role of PI’, ‘Planning Your Research Vision’ and ‘Leading and Managing in an Academic Environment’. The mentors (both at the host institution and overseas) will be instrumental in helping to further the PI’s leadership skills.
Research Skills
To plan the development of research skills, the PI and mentor have devised a training needs analysis and an associated training program to meet the needs of the project and future career aims. The analysis identified strengths in spatial analysis, computational modelling and writing funding applications. The main development areas identified were in spatial (and non-spatial) statistics, data mining, cluster analysis as well as research impact and knowledge exchange. To develop skills in these areas, the PI will take advantage of his British Academy Skills Acquisition grant to visit Prof. Chris Brunsdon (University of Liverpool) to consolidate existing experience in spatial statistics and develop a new method for crime hotspot analysis. The plan also developed a programme of work offered internally by SDDU and three specific courses hosted externally: Data Mining in R; Cluster Analysis; and From Research to Impact.
On top of these research skills, the PI will continue to develop teaching skills by completing the ULTA-2 qualification to move from an HEA Associate to a Fellow (Professional Standard SD2).
Knowledge Exchange
This research project will help the PI to develop his existing skills in translating knowledge to academic and non-academic audiences. He has an existing track record in bridging the gap between policy makers and academia, including ongoing crime simulation work with West Yorkshire Police and Leeds City Council, burgeoning collaborations with Telefónica UK, organising practitioner workshops (through the GeoCrimeData project), debates in non-academic outlets (Arthur, 2010) and presentations at practitioner-oriented conferences (such as the National Crime Mapping Conference). This project will allow the PI to build on these experiences through a series of user engagement activities and customised outputs, as discussed in Pathways to Impact. The numerous visits to external organisations will also foster academic knowledge exchange and the PI will be proactive in presenting at hosts’ seminar programmes. To formally build knowledge exchange skills, the PI will undertake a Media Training course offered by the ESRC, as well as courses hosted at Leeds SDDU. The PI will also engage with the Talisman NCRM node to impart knowledge through training events.